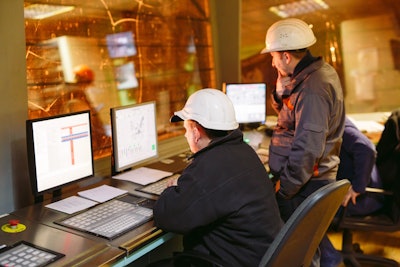
Over the last few years as Artificial Intelligence, machine learning, and other algorithmic tools have risen to greater use and prominence, the biggest question to me has been how these types of tools might be put to use in the production, mix design, and quality control of asphalt production. Large scale data capture was already seeing adoption, and the rollout of Environmental Product Declarations (EPDs) has only spurned the need for high fidelity production data and analytics.
While there's certainly a present consensus that the human element should never be fully eliminated, it doesn't seem that far fetched or that far off into the future where high-functioning AI asphalt mix assistants could be trained on a plant's own internal data sets, providing acute and long-term decision making. Certain experts in the industry agree with that sentiment.
"AI asphalt production assistants that use a plant's own data sets could be developed within the next 18 months, as technology and data handling capabilities continue to improve," said Gary Bisel, Product Owner at Command Alkon. "The evolution depends significantly on advancements in AI algorithms tailored for specific operational processes within the asphalt industry." Command Alkon recently released a unified, AI-powered platform called Command Cloud.
In some ways it's alarming to think of such powerful tools being available, in potentially less that two year, but, as is the case with technological advancement in any space, there are barriers and developmental hurdles that must be overcome. But what would those look like in the realm of our industry?
"The main barriers to developing AI tools for asphalt production include the need for large, high-quality datasets, significant investment in technology infrastructure, and working that learning into a simple user experience that empowers plant personnel to produce the best asphalt possible," Bisel said.
Let's break down these barriers and what they mean:
- Large, high quality datasets
- Technology infrastructure investments
- User interface and workability
The first barrier is likely the easiest to overcome, or, at the very least, the one to be overcome first. AI's thirst for data is never-ending. There will never be "enough" data, but, rather, there is a threshold of viability required. What that threshold is, who can say? What we do know is that the more, the better. Thankfully, the industry is gathering large quantities of high-fidelity data in larger numbers than ever before. Some are doing so because of the requirements for filing EPDs. They use tools like the National Asphalt Pavement Associations' Emerald Eco EPD tool (https://asphaltepd.org/), where producers enter their captured plant data.
The demand for this data has only increased the use of newer, digital tools, which have the added benefit of being more efficient and cost effective. What we are hypothesizing here is that, beyond the use of EPDs, all that captured data could also become useful to producers in added ways with the incorporation of AI.
As far as technological infrastructure investments, some of these are out of the hands of the industry directly. They rely on federal contributions and interest, which can change based on the given administration and its political focus. However, the public sector isn't the only source, and when it comes to private investment in artificial intelligence, the United States ranks first in 2024 with an estimated $249 billion1 in private funding, followed by China ($95 Billion), and then the United Kingdom ($21 billion).
The hardest step, seemingly, would be how to take things down to the user level, and deliver a product that is functional, helpful, and profitable. That trio of factors is the potential stumbling block for many AI related tools. The nuance required to create something of this complexity is a lot deeper than just throwing a sufficient amount of money at it. But there's another question at-hand. Many might wonder if all this trouble is even worth it? Humans have been doing it, and will keep doing it, so is there any real benefit here to be gained?
"An AI tool for asphalt production would likely benefit the industry by enhancing precision in mix designs, improving quality control, and optimizing resource use, which can lead to cost savings and environmental benefits," said Bisel. "There are other areas where AI can play a critical role such as logistics optimization and more dynamic pricing that account for operational constraints and customer behaviors. Its success will depend on how easily it integrates into existing systems."
Crossing The Finish Line
It's a long walk with a lot of "ifs" but should all these barriers be overcome, and with the next two years we have AI asphalt tools in the hands of producers, where might the most impact be felt in the process? What aspects of production could see the biggest benefit and optimization? What AI models do best is crunch numbers, analyze, and give predictive measures.
"The aspects of asphalt production that would benefit most from AI include mix design optimization, where AI can analyze data to produce more consistent and higher-quality asphalt, and predictive maintenance, which can minimize downtime by forecasting equipment failures before they occur," said Bisel.
But they are quick to point out that their aspects of the production process that wouldn't really benefit much from AI and automation. Those types of tasks include ones that require high levels of human judgment and adaptation, such as on-site adjustments during asphalt laying.
"These elements often depend on real-time decisions and adjustments that AI infrastructure will need to push towards the edge where man and machine are interacting," concluded Bisel.